by Florian Butollo on 8th April 2020 @flobutTwitterFacebookLinkedin
This series is a partnership with the Weizenbaum Institute for the Networked Society
Continuing our series on artificial intelligence, AI can augment human work—if workers’ representatives have a voice in implementing it.
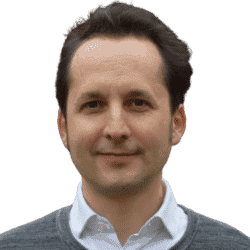
The discourse on artificial intelligence and work is shaped by conflicting narratives. Disempowering notions about mass unemployment and a loss of human control in the face of ever-more-powerful machines are widespread. But AI also inspires visions of human empowerment, according to which labour will be upgraded as machines support human effort and relieve us from the burden of onerous work, leaving us with more interesting, creative and cognitive tasks.
Both narratives are one-sided, deriving projections as to the future of work from the nature of technology as such. To overcome this simplistic dichotomy, the social context in which AI is introduced needs to be addressed. It is not just an interaction between man (or woman) and machine—AI is implemented within a far-flung division of labour, which entails multiple forms of co-operation, task specialisation and inequality. To answer the question of who benefits and who loses through its introduction, it is thus necessary to ask how relations of power between human agents are reconfigured.
Significant limitations
Hubris surrounds the term AI and is responsible for many of the misconceptions. The present technological path of machine learning has generated astonishing breakthroughs, yet significant limitations are encountered when the calculated results are contextualised and applied.
And while it is now possible to detect patterns in massive data sets which surpass the capabilities of human reason—essentially amounting to a different form of intelligence than that of humans—the ‘predictions’ derived from these are structurally conservative. They merely project such patterns into the future, based on correlations established rather than a deeper understanding of underlying factors.
What is more, AI systems continue to be trained towards very specific tasks and cannot transfer capacities to different data sets or changed surroundings. In other words, AI delivers highly-sophisticated statistical evidence for processes of high regularity in controlled surroundings.
There is a multiplicity of applications where these forms of pattern recognition matter, especially in the image or speech recognition and match-making which constitute the main fields of AI today. But this is intelligence in the statistical sense, not anything equivalent to human intelligence.
It fails to work once there are more complex, multi-factor environments involved—think Brexit or the notorious butterfly which might trigger a hurricane in a different region of the world! Human reasoning must step in to contextualise AI results, to understand its implications in real-life scenarios.
Augmented intelligence
In
terms of possible impacts on work, this means AI can be used to
subordinate workers to the mechanical calculations of the machine or to
empower them to contextualise and use AI as augmented human intelligence. Both approaches exist.
The first path isolates the work process from its real-life context. The design of a logistics warehouse or simple manufacturing operation can easily be translated into a data model with input, processing and output variables. AI algorithms can recurrently recalculate the set of factors involved and transmit these to human agents, obliged to follow suit.
Such forms of automated decision-making leave little room for the opinions of workers. Devices displaying the next operation approximate to ‘objective’ efficiency and functionality, to the extent that it becomes futile to argue. The bugs and readjustments that (as always) occur remain the preoccupation of data scientists and management. Workers are supported in their actions but they become highly replaceable, their bargaining power undermined.
The second path ascribes the tasks of contextualising AI to workers. AI might provide transparency about the current state of processes and hints as to possible measures to smooth the operation of a firm, be it a factory or an office. Yet humans face the challenge to interpret such results, based on their capacity to assess the surrounding factors and their experience. This way, decisions can be augmented via a translation and adaption to real-life conditions, building on work experience, intuition and general reasoning. These capacities can be developed through enhancing workers’ capacities to understand, interpret and act upon automated decision-making.
New forms of interaction
It is easy from this to deduce scenarios of a downgrading or an upgrading of work. The point, however, is to identify the variables that affect whether one tendency or the other predominates. This is not rooted in the structural surroundings of certain work contexts or in technology itself but in the active design of new forms of man-machine interaction.
Three dimensions are particularly relevant. The first concerns the fundamental question of investment in technologies, the second the design of interfaces between AI and its users and the third the challenge of equipping workers to upgrade their skills.
Regarding investments, AI can be used for a broad variety of tasks which can be detrimental or supportive when it comes to workers’ empowerment. The question of how technological choices affect power relations in the workplace is a complicated one which needs to move centre-stage in discussions among workers’ representatives. It is linked to management choices favouring the design of enterprises as learning organisms (thus requiring the input of workers) as against neo-Taylorist options that reduce workers to narrowly-circumscribed functions.
Next, the design of technology becomes an important matter for workplace politics. Do the interfaces of AI systems indicate a set of options and the contingency of automatically-generated results? Or do they narrowly prescribe actions that will be mistakenly taken as givens by human agents? Does AI challenge us to interpret its results or relegate us to an observing position? These are delicate questions as to what roles are ascribed to workers in AI models.
Finally, how do companies support workers in developing new skills in a setting of augmented intelligence and how is this incentivised? Calls for more extended training and lifelong learning are widespread—workers need to acquire a deeper understanding of automated processes to make the right decisions, involving the skills to negotiate the translation of insights from the data level to physical processes and real-life communication.
But if workers need to learn more and constantly, how is this to be encouraged? If lifelong learning becomes a requirement that is not compensated through higher wages and relief from other responsibilities, it could soon become not a blessing but a burden. Workers would need to run to stand still in the hierarchies of the workplace.
Tough challenges
All these dimensions constitute tough challenges for workers, works councils and trade unions. They are relevant fields for designing the workplaces of the future, as the technological choices and their embeddedness are surrounded by conflicting interests, in which workers need to strengthen their voice. This necessitates an upgrading of the side of labour towards stronger capabilities in evaluating technologies and putting them to use according to their interests.
And this challenge becomes enduring: AI systems are not merely another machine which once introduced keeps on working in the same way, but learning organisms which modify their functions going forward. AI thus requires an augmentation of bargaining intelligence, so as to be capable of affecting the balance of forces on the shopfloor to workers’ advantage.

—
Source of this article: https://www.socialeurope.eu/